Necdet Aybat
Foundational AI:
- design of new optimization algorithms
- federated learning
- distributed multi-agent optimization
Applied AI Research
- matrix decomposition for image-video processing
Externally Funded AI Projects
- ONR Grant N00014-21-1-2271, “Collaborative Proposal: Robust Primal-Dual Algorithms for Saddle Point Problems with Applications to Multi-Agent Systems”, N. S. Aybat (PI) and M. Gurbuzbalaban (PI), 04/01/2021 - 03/31/2024 ($652,648)
- NSF Grant CMMI-1635106, “Decentralized power flow optimization on electricity grids via distributed consensus methods”, N. S. Aybat (PI), 09/01/2016 - 08/31/2020 ($235,852)
- ARO Grant W911NF-17-1-0298, “Decentralized methods for multi-agent problems over networks,” N. S. Aybat (PI), 07/01/17 - 03/31/18 ($60,000)
- NSF Grant CMMI-1400217, “Resolving Parametric Misspecification: Joint Schemes for Computation and Learning”, U. V. Shanbhag (PI), N. S. Aybat (co-PI), 08/01/2014 - 07/31/2017 ($300,000)
AI-related Courses
- IE/EE 585 Convex Optimization
- IE 597 Modern Data Analytics
Webpage
Related Recent Publications
- E. Yazdandoost Hamedani and N. S. Aybat, “A Primal-Dual Algorithm with Line Search for General Convex-Concave Saddle Point Problems,” SIAM Journal on Optimization (SIOPT), 31(2), 1299–1329 (2021).
- S. Davanloo Tajbakhsh, N. S. Aybat, and Enrique del Castillo, “On the Theoretical Guarantees for Parameter Estimation of Gaussian Random Field Models: A Sparse Precision Matrix Approach” Journal of Machine Learning Research (JMLR), 21(217), 1−41 (2020).
- N. S. Aybat, A. Fallah, M. Gürbüzbalaban, A. Ozdaglar, “Robust Accelerated Gradient Methods for Smooth Strongly Convex Functions,” SIAM Journal on Optimization, 30(1), 717-751 (2019).
- N. S. Aybat, E. Yazdandoost Hamedani, “A Distributed ADMM-like Method for Resource Sharing over Time-varying Directed Networks," SIAM Journal on Optimization, 29(4), 3036–3068 (2019).
- J. Wang, M. Ashour, C. M. Lagoa, N. S. Aybat, H. Che, “A fully distributed traffic allocation algorithm for nonconcave utility maximization in connectionless communication networks," Automatica, 109, 108506 (2019).
- N. S. Aybat, A. Fallah, M. Gürbüzbalaban, A. Ozdaglar, “A Universally Optimal Multistage Accelerated Stochastic Gradient Method,” Advances in Neural Information Processing Systems (NeurIPS), 32, pp. 8523-8534 (2019).
- M. Ashour, C. M. Lagoa, and N. S. Aybat. “Lp Quasi-norm Minimization,” 2019 53rd Asilomar Conference on Signals, Systems, and Computers, IEEE, pp. 726-730 (2019).
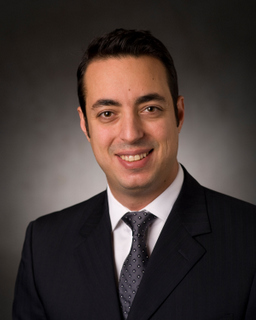
Necdet Aybat
Associate Professor of Industrial and Manufacturing Engineering